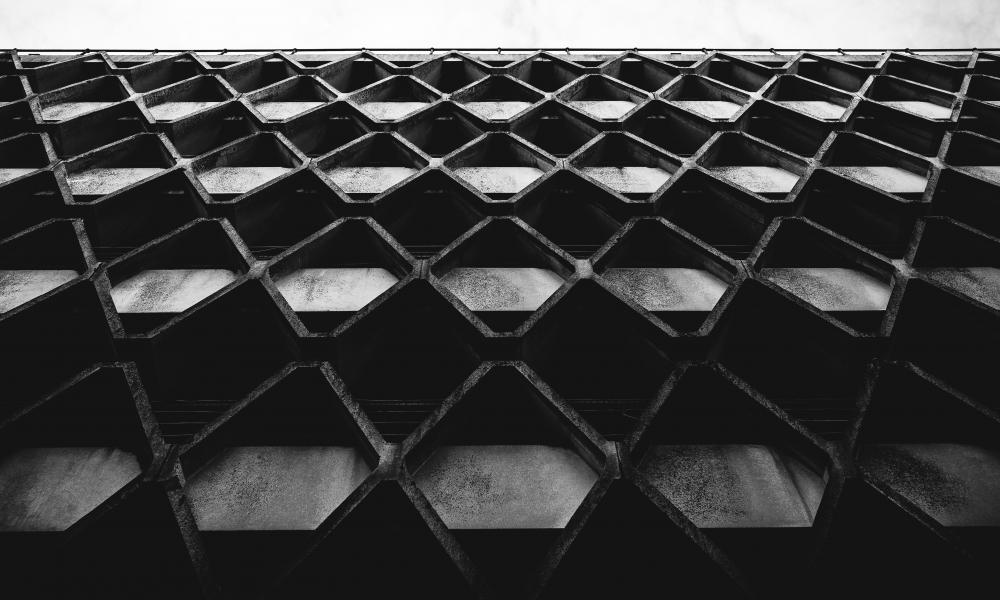
Second post in a series of posts written by members of the Qurit Team. Written by Fereshteh Yousefi Rizi, PhD.
With the growing importance of PET/CT scans in cancer care, the incentives for research to develop methods for PET/CT image enhancement, classification, and segmentation have been increasing in recent years. PET/CT analysis methods have been developed to utilize the functional and metabolic information of PET images and anatomical localization of CT images. Although tumor boundaries in PET and CT images are not always matched [1], in order to use the complementary information of PET and CT modalities, existing PET/CT segmentation methods either process PET and CT images separately or simultaneously to achieve accurate tumor segmentation [2].
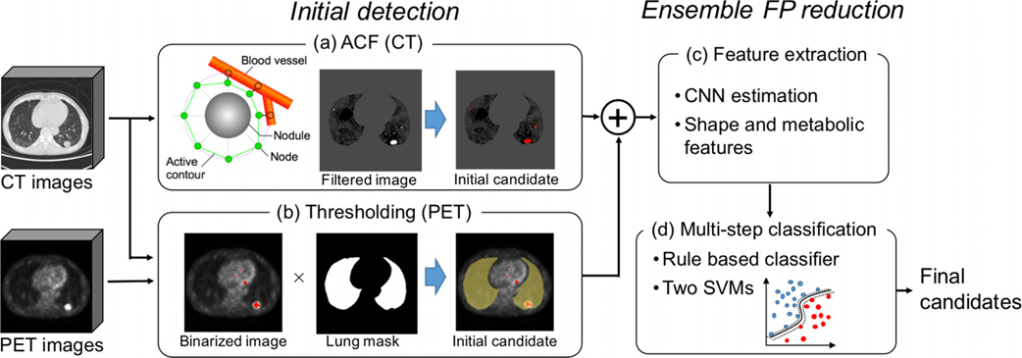
Regarding standardized, reproducible, and automatic PET/CT tumor segmentation, there are still difficulties for clinical applications [1, 3]. The relatively low resolution of PET images as well as noise and possible heterogeneity of tumor regions are limitations to this end. Besides, PET-CT registration (even with hardware registration) may cause some errors due to patient motion [4]. Moreover, fusion of complementary information is problematic for PET and CT images [2, 5]. To address these issues, segmentation techniques in PET/CT images can be categorized into two categories [5]:
- Combining modality-specific features that are extracted separately from PET and CT images [6-9]
- Fusion of complementary features from CT and PET modalities that are prioritized for different tasks [2, 5, 10-13].
Figure 1 depicts overall scheme of a proposed method by Teramoto et al. [6] to separately identify tumor region on the PET and CT images. Another proposed PET/CT co-segmentation method by Zhong et al. [1] is shown in Figure 2 adapted from modality-specific encoder branches.

Deep learning solutions such as V-net [14], W-net [15], and generative adversarial network(GAN) [16] have gained much attention recently for medical image segmentation [17]. Accessing sufficient amount of annotated data, deep models outperform majority of conventional segmentation methods without the need for expert-designed features [1, 18]. Furthermore, there are some ongoing studies on using unsupervised and weakly supervised models [4] or noisy labels [19] and also scarce annotation [20] to make the solutions less dependent on the radiologists.
I am currently working on developing new techniques to improve tumor segmentation results, benefiting from PET/CT complementary information and considering the use of less annotated data, in order to significantly improve predictive models in cancer.
References
1. Zhong, Z., Y. Kim, K. Plichta, B.G. Allen, L. Zhou, J. Buatti, and X. Wu, Simultaneous cosegmentation of tumors in PET-CT images using deep fully convolutional networks. Med Phys, 2019. 46(2): p. 619-633.
2. Li, L., X. Zhao, W. Lu, and S. Tan, Deep learning for variational multimodality tumor segmentation in PET/CT. Neurocomputing, 2019.
3. Markel, D., H. Zaidi, and I. El Naqa, Novel multimodality segmentation using level sets and Jensen‐Rényi divergence. Medical physics, 2013. 40(12): p. 121908.
4. Afshari, S., A. BenTaieb, Z. Mirikharaji, and G. Hamarneh. Weakly supervised fully convolutional network for PET lesion segmentation. in Medical Imaging 2019: Image Processing. 2019. International Society for Optics and Photonics.
5. Kumar, A., M. Fulham, D. Feng, and J. Kim, Co-learning feature fusion maps from PET-CT images of lung cancer. IEEE Transactions on Medical Imaging, 2019. 39(1): p. 204-217.
6. Teramoto, A., H. Fujita, O. Yamamuro, and T. Tamaki, Automated detection of pulmonary nodules in PET/CT images: Ensemble false‐positive reduction using a convolutional neural network technique. Medical physics, 2016. 43(6Part1): p. 2821-2827.
7. Bi, L., J. Kim, A. Kumar, L. Wen, D. Feng, and M. Fulham, Automatic detection and classification of regions of FDG uptake in whole-body PET-CT lymphoma studies. Computerized Medical Imaging and Graphics, 2017. 60: p. 3-10.
8. Xu, L., G. Tetteh, J. Lipkova, Y. Zhao, H. Li, P. Christ, M. Piraud, A. Buck, K. Shi, and B.H. Menze, Automated whole-body bone lesion detection for multiple myeloma on 68Ga-Pentixafor PET/CT imaging using deep learning methods. Contrast media & molecular imaging, 2018. 2018.
9. Han, D., J. Bayouth, Q. Song, A. Taurani, M. Sonka, J. Buatti, and X. Wu. Globally optimal tumor segmentation in PET-CT images: a graph-based co-segmentation method. in Biennial International Conference on Information Processing in Medical Imaging. 2011. Springer.
10. Song, Y., W. Cai, J. Kim, and D.D. Feng, A multistage discriminative model for tumor and lymph node detection in thoracic images. IEEE transactions on Medical Imaging, 2012. 31(5): p. 1061-1075.
11. Bi, L., J. Kim, D. Feng, and M. Fulham. Multi-stage thresholded region classification for whole-body PET-CT lymphoma studies. in International Conference on Medical Image Computing and Computer-Assisted Intervention. 2014. Springer.
12. Kumar, A., J. Kim, L. Wen, M. Fulham, and D. Feng, A graph-based approach for the retrieval of multi-modality medical images. Medical image analysis, 2014. 18(2): p. 330-342.
13. Hatt, M., C. Cheze-le Rest, A. Van Baardwijk, P. Lambin, O. Pradier, and D. Visvikis, Impact of tumor size and tracer uptake heterogeneity in 18F-FDG PET and CT non–small cell lung cancer tumor delineation. Journal of Nuclear Medicine, 2011. 52(11): p. 1690-1697.
14. Milletari, F., N. Navab, and S.-A. Ahmadi. V-net: Fully convolutional neural networks for volumetric medical image segmentation. in 2016 Fourth International Conference on 3D Vision (3DV). 2016. IEEE.
15. Xia, X. and B. Kulis, W-net: A deep model for fully unsupervised image segmentation. arXiv preprint arXiv:1711.08506, 2017.
16. Zhang, X., X. Zhu, N. Zhang, P. Li, and L. Wang. SegGAN: Semantic segmentation with generative adversarial network. in 2018 IEEE Fourth International Conference on Multimedia Big Data (BigMM). 2018. IEEE.
17. Taghanaki, S.A., K. Abhishek, J.P. Cohen, J. Cohen-Adad, and G. Hamarneh, Deep Semantic Segmentation of Natural and Medical Images: A Review. arXiv preprint arXiv:1910.07655, 2019.
18. Zhao, Y., A. Gafita, B. Vollnberg, G. Tetteh, F. Haupt, A. Afshar-Oromieh, B. Menze, M. Eiber, A. Rominger, and K. Shi, Deep neural network for automatic characterization of lesions on 68 Ga-PSMA-11 PET/CT. European journal of nuclear medicine and molecular imaging, 2020. 47(3): p. 603-613.
19. Mirikharaji, Z., Y. Yan, and G. Hamarneh, Learning to segment skin lesions from noisy annotations, in Domain Adaptation and Representation Transfer and Medical Image Learning with Less Labels and Imperfect Data. 2019, Springer. p. 207-215.
20. Tajbakhsh, N., L. Jeyaseelan, Q. Li, J.N. Chiang, Z. Wu, and X. Ding, Embracing imperfect datasets: A review of deep learning solutions for medical image segmentation. Medical Image Analysis, 2020: p. 101693.