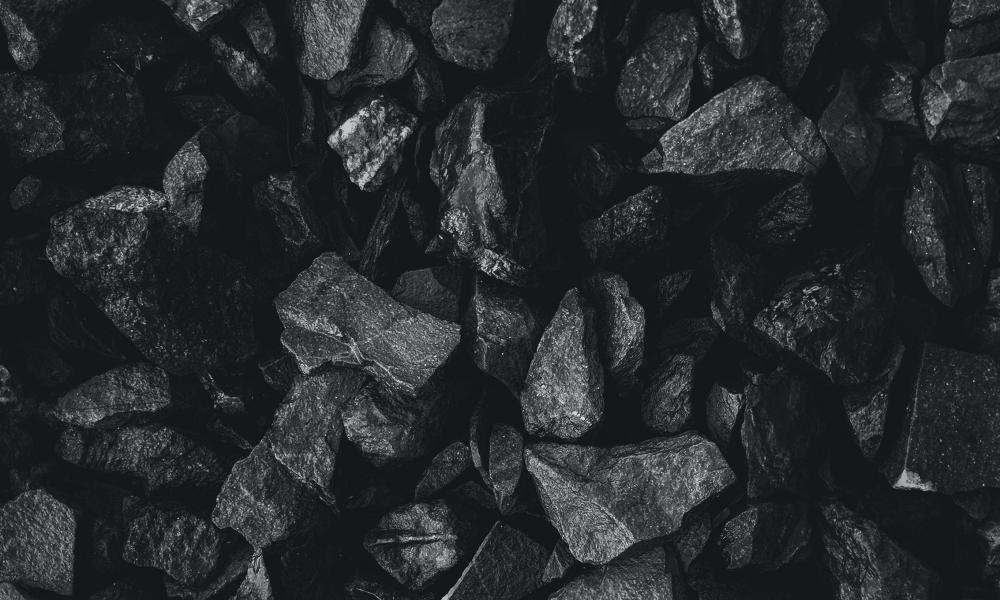
Fourth post in a series of posts written by members of the Qurit Team. Written by Mohammad Salmanpour.
At Qurit lab, my research aim is to subdivide Parkinson’s disease (PD) into specific subtypes. This is important since homogeneous groups of patients are more likely to share genetic and pathological features, enabling potentially earlier disease recognition and more tailored treatment strategies. We aim to identify reproducible PD subtypes using clinical as well as imaging information including radiomics features, via hybrid machine learning (ML) methods that are robust to variations in the number of subjects and features. We also aim to specify most important combination of features for clustering task as well as prediction of outcome.

We are applying multiple hybrid ML methods constructed using: various feature reduction algorithms, clustering evaluation methods, clustering algorithms and classifiers, as applied to longitudinal data from PD patients.

So far, we are seeing that when using no radiomics features, the clusters are not robust to variations in features and samples, whereas utilizing SPECT radiomics information enables consistent generation of 3 distinct clusters, with different motor, non-motor and imaging contributions.

At Qurit lab, we are demonstrating that appropriate hybrid ML and radiomics features enable robust identification of PD sub-types as well as prediction of disease subtypes.